The mix between the risky nature of financial markets and the crucial role of Asset Managers makes AI reliability an important factor to consider when investing. Just as buildings need solid foundations to resist earthquakes, AI models require learning from meaningful data to deliver what they promise: greater portfolio efficiency, risk control and the ability to continuously adapt to and improve over each market cycle.
‍
In this article, we will discuss:
‍
- Why valuable data is so important for AI models in investments
- Which data is best to train AI models for investments
- The Power-Horizon Tradeoff In Financial Data
- The role humans play in training AI models
‍
‍
With Great Power Comes Great Responsibility
‍
‍

‍
In 2002, the first Spider-Man movie made popular the following quote: “With Great Power Comes Great Responsibility”. In the movie, when Peter Parker hears these words from his Uncle Ben, he is still sorting out how to deal with his supernatural powers. However, only after his uncle’s death, Peter fully understands the meaning of this powerful advice, making the decision to become the fair superhero on which New York citizens can rely on.
‍
To some extent, this principle (“With Great Power Comes Great Responsibility”) is true to frame the increasingly relevant role that Artificial Intelligence (AI) is playing in the Asset Management industry. New technologies like AI are allowing investors to better analyse financial data and gain a deeper understanding of the inner dynamics of financial markets: however, the unprecedented power of AI calls for considerable responsibility for those building AI-driven investment solutions.Â
‍
In this article, we will explain the essential factors that are needed to fully unlock the potential of AI in order to build reliable investment solutions for institutional investors. We are going to observe how this requires the most meaningful data along with rigorous supervision of the crucial human factor.
‍
Why Is Data So important For AI Models?
AI models have an unparalleled capacity to analyse data and make accurate predictions, but, if the wrong input data is given, the model could infer wrong or non-existent relationships that will induce to incorrect conclusions. Consequently, the more high-quality data the financial AI model receives, the better its estimations will be. From this standpoint, it is clear why the expression “garbage-in, garbage-out” is an extremely important expression on how to correctly use AI in investments: because financial AI models need data with exceptionally high-quality standards.
‍
Which Data Is Best To Train AI Models For Investments?Â
Input data to train AI models should be accurate and indisputable, in the sense of data on which everyone agrees on. Additionally, data should be truly informative in order to avoid fallacious connections, and false cause-effect relationships. But, if we dig deeper, how can we define what truly informative data is?
‍
The Power-Horizon Tradeoff In Financial Data
Even if we have understood the potential of AI techniques and the importance that data quality has, it is not always clear which type of financial data to actually use. Indeed, today investors find themselves with an immense quantity of financial data to choose from. Undoubtedly, each type of financial data has unique pieces of information, with strengths and weaknesses that are better suited for different objectives.
‍
In fact, if one aims to accurately train an AI model, then input data should be informative in the sense of being relevant for the investment horizon chosen to execute a particular strategy. AI models that aim to exploit the benefits of staying invested in the market over time need to take into account a medium to long-term approach, in order to avoid being affected by temporary short-term shocks. To identify the most effective type of financial data in this sense, the graph below depicts how macro-types of financial data differ in terms of predictive power (vertical axis) and predictive horizon (horizontal axis).
‍
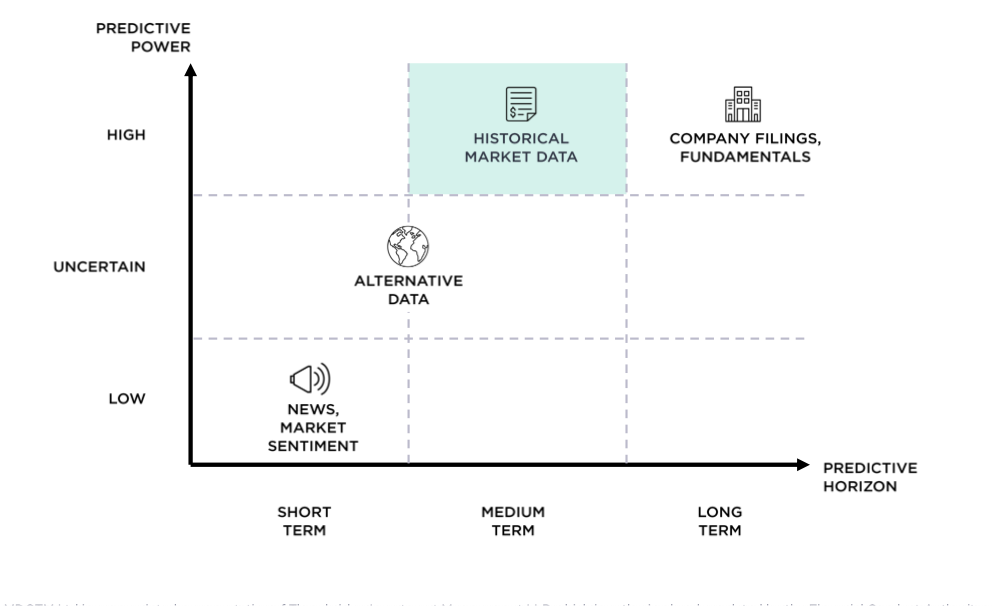
The power-horizon tradeoff: how financial data differs in terms of predictive power and predictive horizon
‍
Predictive power is related to how much essential information is aggregated in the data, or better the cause-effect relationships among assets. Predictive horizon refers to the time it usually takes for that information to be priced into securities and turned into useful investment opportunities.
‍
Therefore, while financial news represents a widely available source of information for many investors, regarding AI, they risk adding information that is only relevant for a short period of time. Equivalently, alternative data only risks adding an extra noise to the analysis that fogs the underlying connections among assets. And, similarly, even though fundamentals have been the main ingredient of long-term value investing, they present structural impediments that restrains AI models from being responsive to current market conditions, and consequently missing investment opportunities. From this standpoint, historical market data emerges as the type of financial data that best balances the predictive power-horizon tradeoff. Indeed, asset prices are a standardised, indisputable and widely-available information source that offers investors the opportunity to have a 360-degree understanding of data.
‍
In fact, historical market data partially embeds all the above-mentioned sources of information, and consequently better reflects the underlying market dynamics. Plus, the information contained in market data seems to be perfectly suited for a medium to long-term horizon, it provides details useful to adapt to the gradual unwrapping of financial markets but avoiding sharp changes in volatility.
‍
Finally, market data perfectly fit the criteria to ensure that AI models function efficiently and correctly: on the one hand, financial markets offer data that is indisputable (once markets close, investors cannot question the price of securities), and, on the other hand, market data is constantly updated. These features greatly ease and improve the prediction power of AI, enabling investors to better extract what mathematician and essayist Nassim Taleb defined in his masterpiece “Fooled by Randomness”: “the signal from the noise”.
‍
Exceptional Data Alone Is Not Enough
Now that we have understood which type of data ensures a correct functioning of financial AI models, we also have to take into consideration that AI models don’t automatically turn data into remarkable investment solutions. Obviously, a well-trained AI model is necessary, and to achieve this, humans play a crucial role.
Human supervision is key when it comes to successfully training an AI model; starting from the data collection, all the way to its continuous learning. As models need to learn which information is correct and which is not, humans’ role is fundamental, because historical market data has to be periodically checked for consistency and possible misconceptions.
‍
Furthermore, an efficient training process is crucial to reduce the risk of overfitting - building overly complex models that tend to make conclusions based on random correlations, mistaking noise for signal. Conversely, well built, trained, and supervised financial AI is capable of building investment strategies that are coherent with investors’ expectations and objectives. If AI is well trained, it can dynamically adapt to current market scenarios and keep volatility under control to deliver intended investment objectives.Â
‍
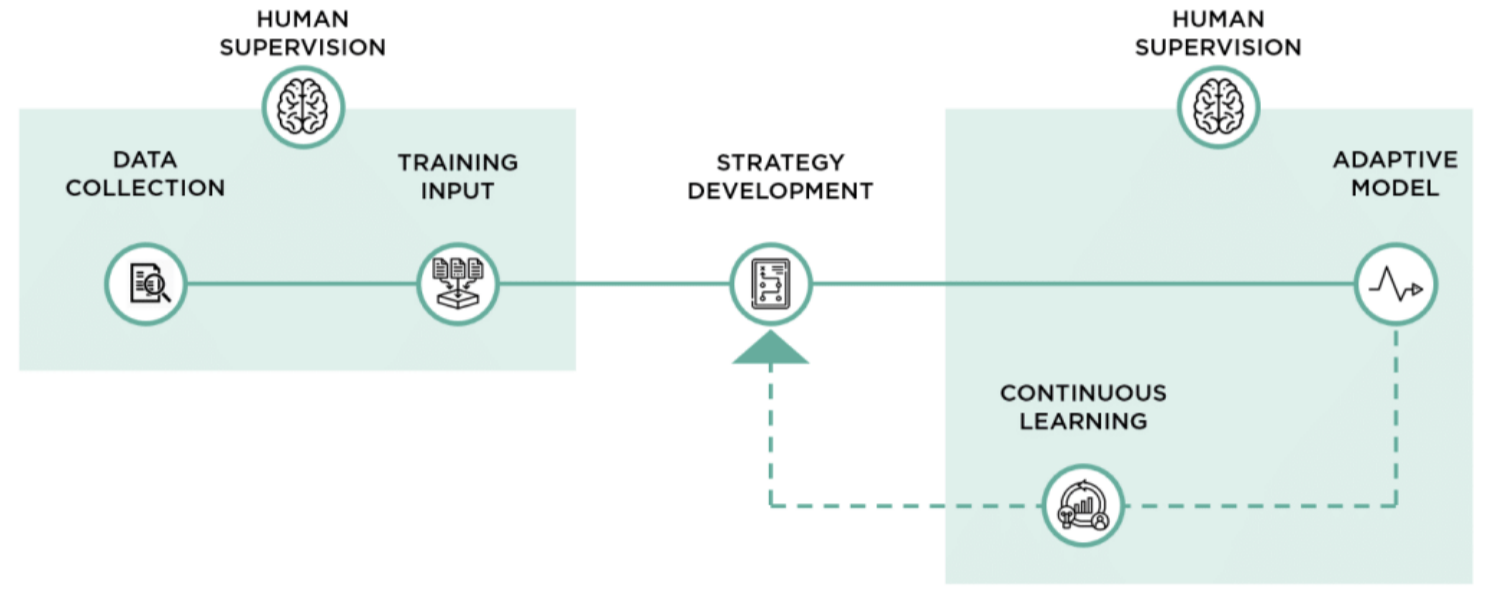
The AI-driven approach: how humans play a vital role in the AI training process
‍
With The Right Data Comes Great Opportunities
AI is not a technology that can magically turn data into a professional investment strategy. However, if reliably trained, it can be a powerful tool to deliver investment results that are meaningful to the investors’ expectations. In this sense, the first step towards unlocking the potential AI in investing consists in using meaningful data.Â
‍
Input data should be standardised, indisputable, and informative in the sense that, to deliver reliable results for institutional investors, input data should possess the highest tradeoff between predictive horizon and predictive power. Historical market data and the metrics that can be calculated from them (variances, covariances, and return distributions) covers these characteristics completely. With the best data, the full benefits of AI in investing can come to light. In the end, meaningful data to train AI models represents a crucial step to deliver exceptional investment strategies – and if properly trained, AI can truly be a powerful tool that builds robust strategies that investors can trust, and successfully exploit.Â
‍
‍
---
‍
Want to know more about how our technology Sphere uses data to provide institutional investors with unbiased, reliable and forward-looking market inputs?
Download the White Paper https://bit.ly/3NdZzS9
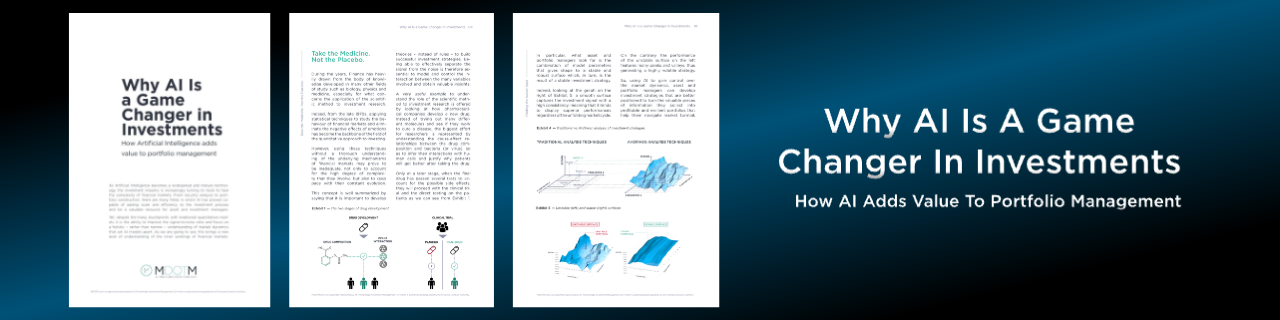
‍
‍
‍
‍
‍