In the world of AI investments, the importance of explainability and reliability cannot be overstated. It's not merely a feature; it's a fundamental principle that brings trust, comprehensiveness, and effective decision-making. At MDOTM Ltd, we've fully embraced this principle. In this research article, we delve into how we ensure AI explainability and reliability in our investment model.
Explainable AI For Investments in Practice
To fully unlock AI's potential in investment decisions, two crucial factors come into play: the utilisation of meaningful data and an understanding of the technology's architecture. In our AI platform called Sphere, these elements are seamlessly integrated to deliver a clear and concise framework for AI explainability.
Utilising Meaningful Data
One of the primary principles of AI explainability is the utilisation of meaningful data. In Sphere's case, this is exemplified through its daily analysis of macro, fundamental, and market data. This rigorous data analysis serves a dual purpose. Firstly, it helps avoid fallacious connections, where correlations are mistaken for causation, ensuring that investment decisions are based on sound reasoning. Secondly, it provides a comprehensive view of the financial landscape, enabling Sphere to make well-informed, forward-looking investment insights.
In an era where data is abundant, Sphere's commitment to data quality stands out. We recognize the significance of accurate and reliable data in making informed investment decisions. By sifting through diverse datasets, we ensure that only the most valuable, quality data is incorporated within the investment model.
An Explainable Architecture by Design
The second core principle of AI explainability lies in having an architecture that is inherently explainable. Sphere's architecture is meticulously designed with this in mind. Every component within Sphere is traceable and explainable, which means that the rationale behind every AI-driven decision is always within reach.
This transparency in architecture serves multiple purposes. It empowers our clients to comprehend the technology's reasoning behind investment insights, ensuring that every recommendation aligns with their investment objectives. It also facilitates trust in AI-driven decisions, as one can trace the path from data to recommendation, fostering a sense of confidence in the decision-making process.
MDOTM's Investment Technology
Our model combines two fundamental components that drive human decision-making: experience and data evaluation. Let's delve into how our investment model reasons, ensuring that every investment decision is made with precision.
To understand the approach that guides our investment decisions, it's important to first examine how human decision-making works. Experienced portfolio managers, in particular, rely on two key components: their past experiences and a thorough evaluation of data.
When faced with the task of making an investment decision, a portfolio manager often begins by drawing from their experience. They recollect past market phases, market dynamics, and even significant black swan events. This experiential knowledge is crucial, as it forms the basis for making educated guesses about the future.
The second component of human decision-making involves data evaluation. In this phase, portfolio managers scrutinize the data to gain a comprehensive understanding of the current market scenario. This data-driven approach is aimed at obtaining the best possible insight in regard to the financial landscape.
Artificial Intelligence also leverages both experience and data evaluation but with a distinct methodology. AI achieves this by harnessing the power of data. This approach complements the traditional/fundamental approach taken by humans. While humans excel when information is scarce and relies on a heuristic approach, AI thrives when faced with abundant information, especially when it entails connecting and analysing a vast number of data points.
MDOTM's investment technology uses a proprietary AI methodology that seamlessly integrates the components of experience and data evaluation:
Non-Chronological Learning:
This pivotal component of our AI model begins with the process of learning from past data, moving beyond simple memorisation of historical dynamics and events. Instead, it focuses on learning the underlying market properties of past events, a concept we refer to as Generalisation. The model delves deeper into the essence of past scenarios, gaining the ability to recognize patterns and similarities among various events. This profound understanding enables the model to adapt effectively to new, previously unseen data and make accurate forecasts.
To explain this, consider the way humans learn to identify an apple tree. We start by looking at various trees and observing their distinct characteristics. We categorize these characteristics, creating unwritten rules that enable us to identify and classify different types of trees. These rules are formed based on features like the shape of leaves, the size of the crown, and the appearance of flowers and fruits. Over time, we create clusters of similar characteristics, making it easier to recognise different tree types.
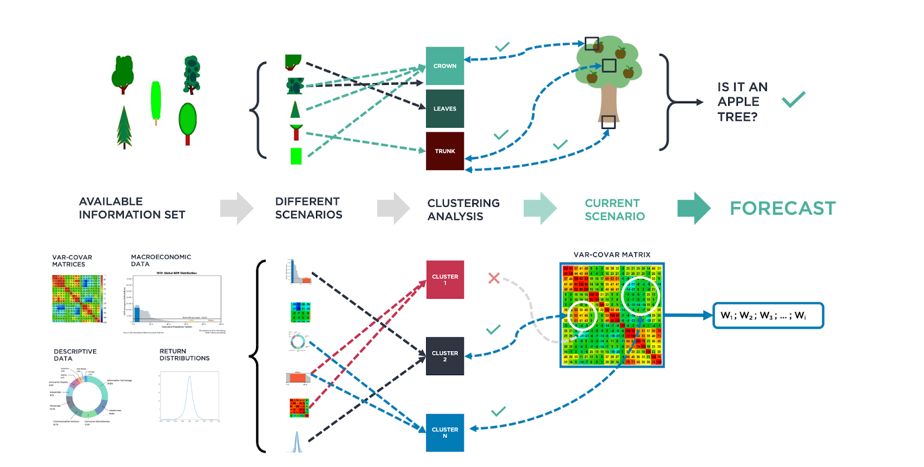
Similarly, MDOTM's AI models use neural networks to observe past market scenarios and cluster them into groups of analogous information. When faced with a new scenario, the model recalls its learning process and generates forecasts for correlations, volatility, and asset risk, while also evaluating the riskiness of various asset classes. These forecasts are based on a non-linear combination of past experiences. By systematically discounting possible changes in variance-covariance matrices and market conditions, the neural network is trained to optimise portfolio efficiency, maximising returns and minimising volatility with each rebalance.
Regime Analysis:
Financial markets operate in specific regimes, characterised by the dynamics, conditions, and behaviour of different components and variables. In an increasingly complex financial landscape, it's vital to be "regime-aware" when making investment decisions. This allows for better positioning of the portfolio's risk, depending on the current market regime.
MDOTM's Market Regime Analysis employs a derivation of Hidden Markov Models (HMM), an unsupervised machine learning technique. This approach is typically used to estimate forward-looking probabilities and understand the evolution of complex systems that cannot be directly observed. HMM helps identify market regimes in terms of risk environment and data coherence, offering a holistic view beyond just return and growth measurements.
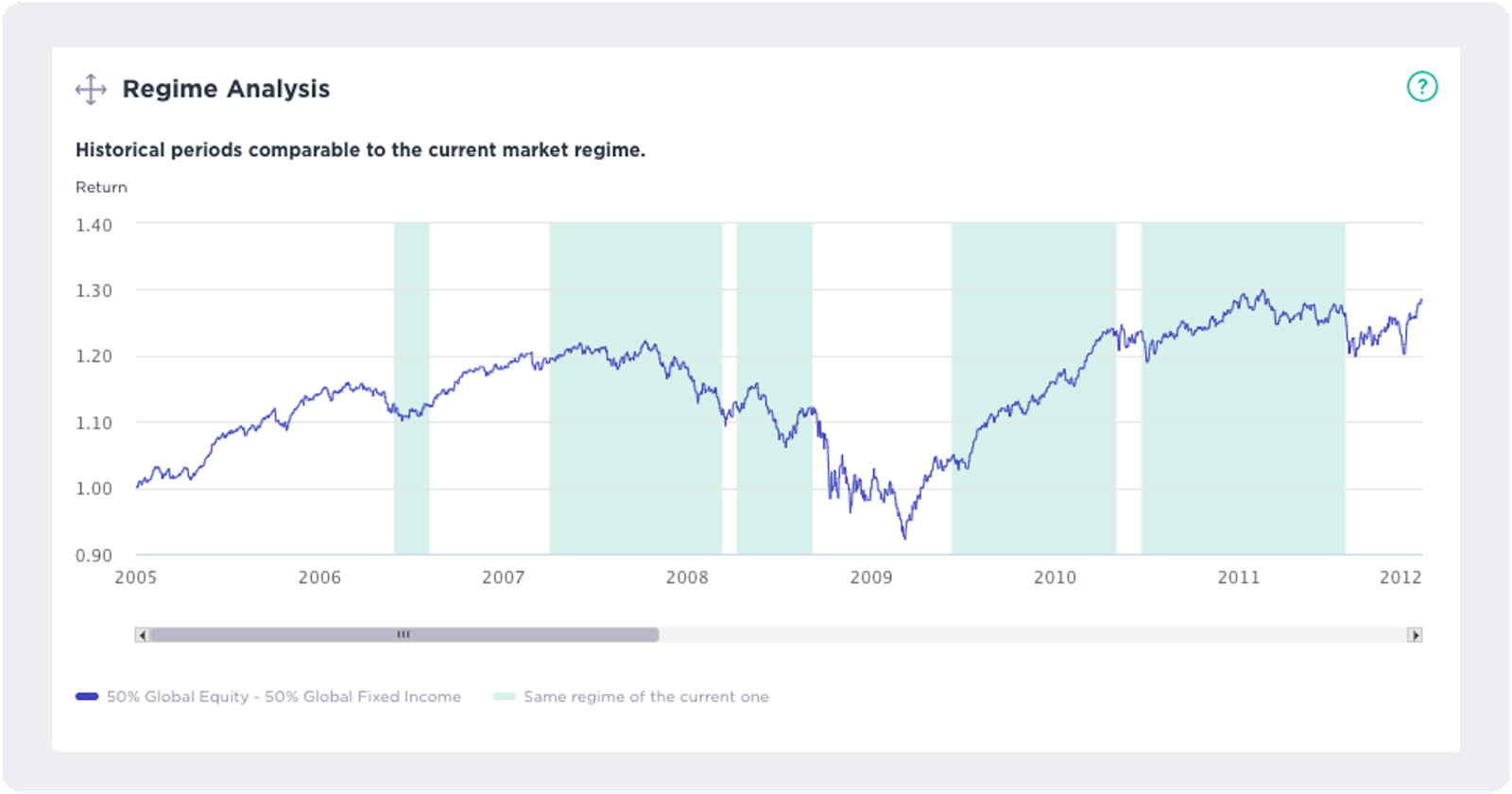
The regime analysis identifies various regimes in the market, each characterised by specific risk conditions and behaviour. The model examines the current market structure, compares it to historical market regimes, and assesses the current market's regime and its expected duration. The technology also looks ahead to determine the probability of each market regime occurring in the near future and the associated transition probabilities. Depending on the market phase identified by HMM, the model generates signals that influence positioning decisions.
MDOTM's investment technology combines Non-Chronological Learning and Regime Analysis to create probabilistic forecasts for key portfolio components, such as expected returns and var-covar matrices. It also incorporates a risk management layer into the investment methodology itself, ensuring that it's an integral part of every decision rather than an afterthought.
Curious To Try Our AI Live?
Book your custom, free demo below to learn how our AI can help you drive results and increase productivity. 👇
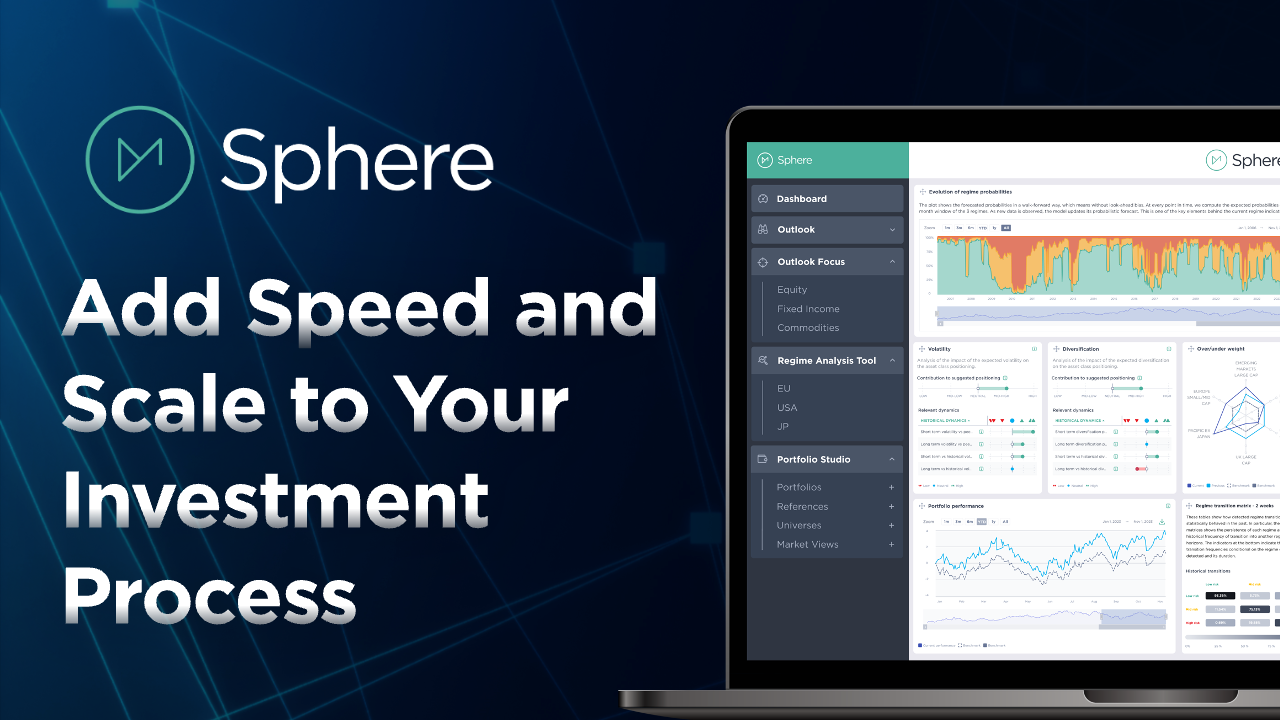
The Significance of Data Quality in Investments
Data forms the base of AI models, and its quality plays a pivotal role in shaping the efficacy of financial AI models. The concept "garbage-in, garbage-out" holds true in the world of AI in investments. Here's why data quality is paramount, and which data is best suited to train AI models for investments.
AI models are renowned for their data analysis capabilities and their ability to make precise forecasts. However, if these models are fed with the wrong input data, they may infer erroneous or non-existent relationships, leading to incorrect conclusions. In essence, the quality of data directly impacts the quality of the AI model's estimations. The importance of data quality is encapsulated in the phrase "garbage-in, garbage-out," highlighting the need for exceptionally high-quality standards in financial AI models.
To train AI models effectively, input data must meet certain criteria. First and foremost, it should be accurate and universally accepted, with a consensus among experts in the field. Additionally, data should be genuinely informative, devoid of fallacious connections and false cause-effect relationships. But what defines truly informative data?
The Power-Horizon Tradeoff in Financial Data
Choosing the right financial data for AI models can be challenging in a landscape inundated with vast amounts of information. Different types of financial data offer unique pieces of information, each with its strengths and weaknesses, making them more suitable for specific objectives.
For AI models intended to capture the benefits of long-term market participation, it's crucial to use data that aligns with the chosen investment horizon. The graph below illustrates the tradeoff between predictive power and predictive horizon of various macro-types of financial data:

Predictive power is the measure of how much essential information is encapsulated in the data, particularly the cause-effect relationships among assets. The predictive horizon relates to the time it typically takes for this information to be reflected in securities prices and become viable investment opportunities.
Financial News: While widely available and frequently used, financial news may introduce information that's only relevant for the short term, potentially causing undue market noise.
Alternative Data: Using alternative data sources can complicate the analysis, as they may introduce noise that obscures the underlying connections among assets.
Fundamentals: While fundamentals are essential for long-term value investing, they have limitations that impede AI models' responsiveness to current market conditions. They may miss short-term investment opportunities.
Historical market data emerges as the data type that strikes the best balance between predictive power and predictive horizon. Asset prices represent standardised, indisputable, and widely available information sources that provide a comprehensive understanding of the market dynamics. The information contained in market data is well-suited for medium to long-term investment horizons, allowing investors to adapt to gradual market developments while avoiding abrupt volatility changes.
Market data excels in two critical areas that ensure the efficiency and accuracy of AI models. Firstly, financial markets provide data that is indisputable - once markets close, the prices of securities are fixed and cannot be questioned. This stability enhances the predictive power of AI, allowing it to discern the signal from the noise.
However, it's essential to understand that while exceptional data is a necessary foundation for financial AI models, it's not sufficient to guarantee outstanding investment solutions. The human element remains integral throughout the AI training process. Humans are responsible for data collection and its ongoing quality control. They ensure that historical market data is periodically checked for consistency and potential misconceptions.
Moreover, a well-structured and supervised AI model is necessary to prevent overfitting - a situation where the model becomes overly complex and generates conclusions based on random correlations, mistaking noise for the actual signal. With proper training, well-constructed financial AI models can develop investment strategies that align with investors' expectations and goals. These models can dynamically adapt to changing market conditions, manage volatility effectively, and deliver the intended investment objectives.
AI, when reliably trained, becomes a powerful tool for generating meaningful investment strategies. However, the first step in harnessing the potential of AI in investments lies in using data that is meaningful. The data should be universally accepted and informative, with the optimal tradeoff between predictive horizon and predictive power. With the right data, AI can unlock exceptional investment opportunities and build robust, trustworthy strategies that investors can successfully rely on. It's the combination of exceptional data and reliable training that empowers AI to deliver remarkable investment results.
The Nexus Between Generative and Analytical AI
In investments, two distinct yet complementary branches of AI have taken centre stage: Analytical AI and Generative AI. These two facets of AI bring unique qualities to the table, and their combination is reshaping investment decision-making.
Analytical AI is the technology that employs machine learning techniques to sift through vast datasets. Its primary mission is to extract hidden insights, identify novel patterns, and establish relationships within datasets. Operating on a foundation deeply rooted in statistics and mathematics, analytical AI utilises methodologies like Linear Regression, Neural Networks, Decision Trees, and Random Forests to accomplish its tasks.
In the financial landscape, MDOTM's investment platform, Sphere, is a prime example of Analytical AI at work. Sphere meticulously analyses millions of historical, market, and macro data points. This deep and advanced analysis brings forth hidden signals within the data noise, providing investment professionals with invaluable insights to guide their investment decisions.
Generative AI stands apart with a wholly different architecture compared to analytical AI. Generative AI models take the input data and learn its patterns and structure, generating entirely new data with similar characteristics. Additionally, Generative AI often involves human interaction through specific prompts or requests.
Take, for instance, Midjourney, an AI-powered image generation tool. Users interact with Midjourney by providing textual or visual prompts, like a description of the image they wish to see or an existing image as a reference. Generative AI, such as Midjourney, then crafts a unique image based solely on the user's input.
Analytical AI and Generative AI can work collaboratively to enhance the way investment decisions are made and understood. Analytical AI provides a data-driven foundation, uncovering trends, correlations, and patterns, giving investment professionals actionable insights.
Generative AI, on the other hand, plays a crucial role in ensuring transparency and explainability in investment decisions. It bridges the gap between the analytical output of AI and the human understanding of the technology’s reasoning. This is especially important in investments, as it empowers investment professionals to make informed decisions and communicate the rationale behind their choices effectively.
Ask Sphere AI
The combination of these two forms of AI takes a significant step forward with the introduction of Ask Sphere AI. This ChatGPT-powered copilot, residing within Sphere, takes on the role of analysing the extensive proprietary data generated and computed by Sphere regarding users' portfolios. By leveraging this vast amount of data, Ask Sphere AI empowers clients to engage with their portfolio data in a transformative way.
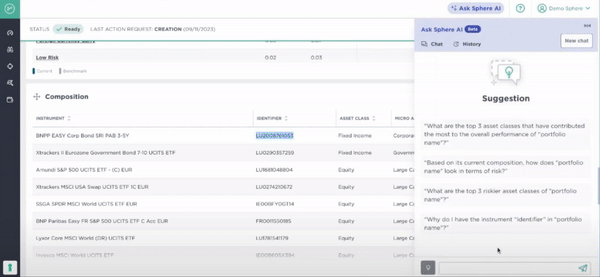
With Ask Sphere AI, clients can delve deeper into their portfolios, gaining real-time insights and answers to their queries, ultimately enhancing their understanding of their investments. The fusion of ChatGPT's natural language processing capabilities with Sphere's data-driven insights provides an exceptional feature for investment managers to interact with their portfolios in a more intuitive and friendly manner.
This collaboration demonstrates Sphere's commitment to enhancing AI explainability and enabling investment professionals to make more informed decisions. Investment managers can seek real-time answers to questions and make more informed decisions, thanks to this feature.
As the investment landscape continues to evolve, the adoption of AI platforms such as Sphere is not just a choice; it is an essential strategic imperative for modern asset management. With Sphere, investment professionals can easily optimize their portfolios, and have a new level of customisation, advanced analysis, and enhanced explainability.
The nexus between generative and analytical AI is reshaping the modern approach toward investment management. Analytical AI's data-driven capabilities are harnessed to uncover hidden insights and optimise portfolios, while Generative AI ensures these insights are translated into clear and comprehensible explanations. Investment professionals can now navigate the dynamic and competitive financial markets with greater precision and confidence, thanks to this collaboration of advanced AI technologies found within Sphere.