For over thirty years, banks, insurance companies, pension funds, hedge funds and investment funds have been using mathematics and statistics on a daily basis to decide how to invest. In this context, the arrival and growing adoption of Artificial Intelligence (AI) models within the investment process officially marks the transition from “data-driven” to an era of "assisted decision-making": or better, the ability to receive recommendations, suggestions and interact dynamically with data, with the benefit of reaching more informed decisions and limiting biases.

How traditional and AI-driven models look at market dynamics
Why is everybody talking about AI today?
According to IBM's estimates, about 90% of the data in circulation have been produced in the last two years. From this point of view, it seems almost consequential to see how in the asset management industry, that has been using statistical techniques and econometric models for decades, all this has had an impact on the way investors look at the markets and make investment decisions.
Recent studies show that the interest of financial institutions in the adoption of new technologies, including AI, is growing strongly. For many institutional investors, banks, insurance companies, funds but also Wealth & Asset managers, investing in technology has become a key element within their strategies to consolidate or acquire a competitive advantage. Among the reasons that drive this acceleration is above all the incremental character of the benefits (in terms of productivity and efficiency) of new technologies.
In the case of AI in the investment process we talk about carrying out increasingly precise and adaptive analysis to changes, allowing asset managers to formulate decisions based on a deeper understanding of the dynamics of financial markets. Unlike the traditional Data-Driven approach that was based entirely on Big Data analysis, the use of AI opens up a more modern era based on Assisted Decision-Making: or better, of investment choices assisted by technology for constant monitoring of risks to a dynamic analysis of correlations and diversification between asset classes.
From this point of view, the new generation of AI-driven models has been able to overcome the obstacles that have limited the use of traditional quantitative methods: including the commonly static approach unable to withstand continuous changes. While, by learning from the data, AI models allow greater flexibility in adapting to new situations, increasing flexibility and adaptability in investment decisions.
In fact, new AI models notably differ from traditional models as to how they address problem-solving. In particular, instead of programming a machine to perform a sequence of consecutive tasks, like traditional algorithms, machine learning algorithms are designed to learn the solution from vast sets of data. This makes them applicable to problems which are not only highly complex, but give rise to high-dimensional datasets and ever-changing dynamics, as it is outlined in the table below.
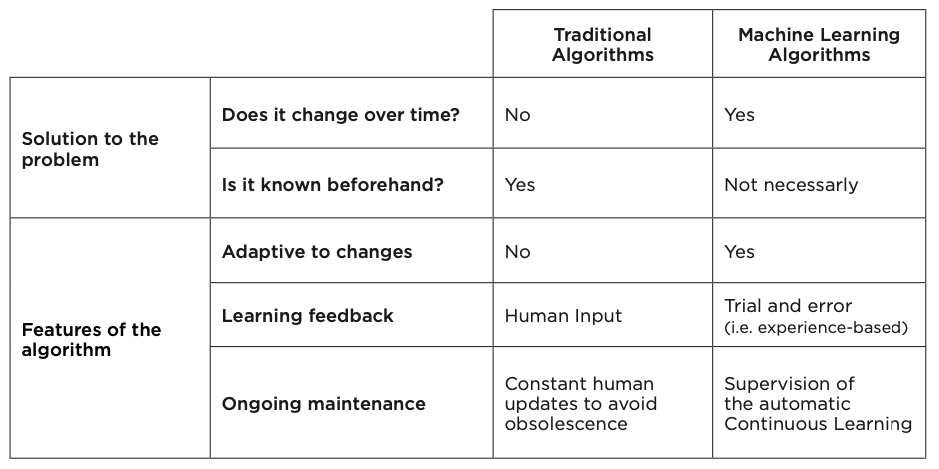
Main differences between machine learning and traditional algorithms
Therefore, if the solution to a problem is known beforehand, a traditional algorithm and a machine learning algorithm would return the same solution in the long run. And, if we were to solve a known problem with machine learning, the solution would eventually be detected as more data is fed into the system. This leads us to an important observation: the advantage of AI models is that they can solve problems without the knowledge of the variables necessary to its respective solution. This is especially relevant for problems that require an intelligent way of making sense of the volume of data collected, just like in the investment process.
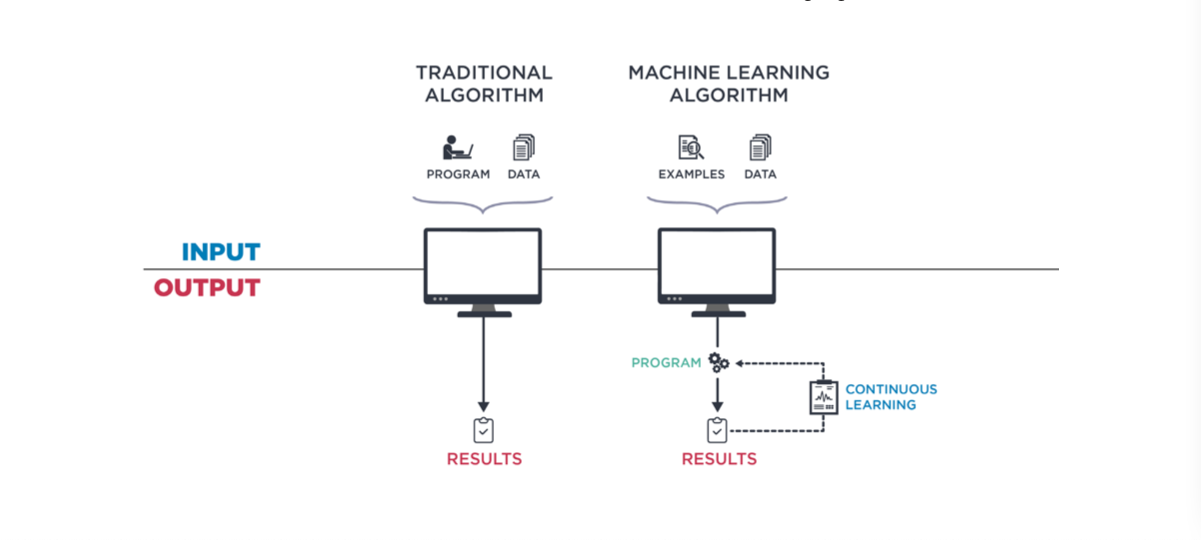
Main differences between a traditional and machine learning algorithm
AI, a Mature Technology
On a deeper level, it seems that the real challenge of an asset manager has become to distinguish the relevant information from the irrelevant one. In this sense, Investment Management, in particular, has been an area that has benefited from the introduction of AI.
Tailor-made portfolio construction, advanced analysis of stocks and risk factors, analysis of outliers and opportunities in terms of tactical and strategic Asset Allocation, are some of the examples of the areas in which AI has found greater application, allowing to grasp details previously hidden in the noise of the markets.
To this extent, the famous strategic consulting firm Gartner every year creates a scale that measures the level of maturity reached by a certain technology. Today, the most mature techniques in the field of AI are Predictive Analytics, these being tools and models that learn to understand the context, create scenarios, suggest alternatives and support decisions, thus allowing assisted decision-making. Immersed in the financial environment, a new generation of tools that help us answer questions such as: Does my portfolio reflect my view of Asset Allocation? What scenarios and risk factors I am most exposed to? And what alternatives do I have to rebalance my portfolio in a rising rate environment?

Maturity achieved in the different branches of Data Science and Artificial Intelligence
Source: Gartner
Assisted-decision making, a crucial opportunity for investment professionals
Especially in investment management, more and more managers consider new technologies, such as AI, a pivotal element to satisfy a more demanding clientele. Assisted Decision-Making is allowing investment managers to remain the main character of the definition of the universe and the execution of the investment strategy, benefiting from the AI’s inputs for portfolio construction. In an industry that for years has been using mathematics and statistics to evaluate investment decisions, AI models are allowing for a more elastic and adaptive way to analyse data, facilitating the transition from Data-Driven to Assisted Decision Making. The ability to see 360 degrees, a new interaction between managers and data, and more informed decisions are the result of these applications. An advantage, which in this context translates into an advantage in terms of performance, risk management and alignment of the portfolio with the evolution of financial markets.