Executive Summary
- AI-based tools like ChatGPT are revolutionising various industries, and the investment sector is no exception.
- AI assists in data analysis, identifying investment signals and providing forward-looking investment inputs.
- Generative and analytical AI can work together to enhance investment decision-making.
- The 4 stages of AI maturity include Base Research, Applied Research, Custom Integrations, and Effective Integration.

How AI is Paving the Way in Investments
The recent explosion of AI, easily demonstrated by the launch of ChatGPT-4, signifies that the widespread adoption of AI is showing no signs of slowing down. Over the past decade, industries like Healthcare, Aviation, Space Exploration, and Automotive have paved the way for AI, showcasing its potential to enhance decision-making processes. The investment industry has taken note and is already leveraging AI's potential to power its decision-making.
The financial market landscape has transformed significantly over the past few decades. Rapid growth in data volume and complexity has reshaped the industry, making it increasingly challenging for investment professionals to identify meaningful insights. As technology evolved and data surged, financial markets grew more intricate, posing substantial challenges to institutional investors. In this intricate environment, the ability to analyse vast and diverse datasets has become essential to making more informed investment decisions and gaining a competitive edge.
AI steps into this complexity as a valuable ally. In various industries, AI has already demonstrated its effectiveness in data analysis. In healthcare, AI assists in the analysis of complex medical data, aiding in diagnosis and anticipating patient outcomes. In the automotive sector, AI processes vast amounts of data from sensors and connected cars, improving vehicle safety and optimising performance.
The investment industry, in particular, stands to benefit significantly from AI adoption. Institutional investors need to analyse enormous volumes of data daily, and AI-based tools offer a way to extract insights efficiently, providing forward-looking analysis and more accurate forecastings.
Consider the use of Neural Networks, which are AI algorithms inspired by the human brain's structure and function. They can identify relationships between different economic indicators and stock market performance, aiding in forecasting market reactions to economic changes.
Another potent AI-based technology is Hidden Markov Models (HMMs). These statistical models excel at analysing and predicting complex patterns in time-series data, making them invaluable for forecasting market trends and identifying investment opportunities, especially in situations where the underlying data-generating system is complex and not directly observable.
These are just a few examples of how AI technologies have evolved into valuable tools for professionals, particularly institutional investors, seeking to maintain a competitive edge in a complex and rapidly changing landscape.
Today, we live in an era of AI democratisation, where the power of AI is accessible to a broader audience than ever before. Unlike in the past, when AI development and deployment were the domain of specialised players, the emergence of cloud-based platforms, open-source tools, and AI platforms has democratised the access to AI. This shift has brought a new wave of innovation, enabling individuals and organisations of all kinds to harness AI's capabilities for a wide array of uses.
Just as airplanes revolutionised travel, AI is transforming how we work, live, and interact with the world around us. Take ChatGPT, for example, which has revolutionised modern work by offering advanced natural language processing capabilities. It can generate human-like responses to queries, providing context-based solutions, and enhancing efficiency, and accuracy across various industries. The latest version of ChatGPT, recently unveiled by OpenAI, takes productivity to new heights, from turning sketches into webpages to creating data visualisations in record time.
Another relevant example is Midjourney, an AI image-generation tool that uses Machine Learning to create unique images based on human inputs. These innovations exemplify AI democratisation, making powerful AI tools accessible to a broader user base.
In the investment industry, a similar democratisation is unfolding, offering investment professionals powerful tools to analyse and interpret financial data with ease. MDOTM's Sphere, for instance, is an AI platform that leverages AI to provide unbiased investment insights and portfolio management.
Sphere empowers institutional investors to combine their expertise with state-of-the-art AI, creating forward-looking investment portfolios for every market scenario. By analysing market, fundamental, and macroeconomic data daily, this AI platform offers unbiased market views across asset classes, geographies, and sectors, supporting asset allocation and portfolio management. Just as ChatGPT transformed information gathering, Sphere democratises access to powerful investment and market insights, revolutionising investment decision-making.
Modern AI Applications in Investment Management
Tasks once considered impossible, such as facial recognition and assisted medical imaging, have become realities thanks to AI models that learn from data, enabling them to comprehend and predict complex systems' behaviours. As previously mentioned, the investment landscape has not remained untouched by this transformative wave. As financial markets grow increasingly complex and competitive, the ability to extract valuable insights from the noise of data has become more critical than ever.
The exponential growth of computing power, famously described by Moore's Law, made AI model training more efficient and cost-effective. As a result, AI transitioned from the confines of computer science labs into the daily workflows of billions of people. The AI that emerged was different from the one often depicted in science fiction; it was more focused and reliable.
AI's influence extends beyond space exploration, benefitting various corporate processes within large institutions across sectors such as automotive, healthcare, pharmaceuticals, high-tech, telecom, and investments. A recent study by Stanford, in collaboration with Bloomberg, Google, and McKinsey, identifies processes, risk management, and product innovation as the areas where AI adoption is most pronounced. By automating low-value tasks, AI allows humans to focus on high-value activities, significantly boosting performance and revenue.
Applications and AI Adoption Levels in Different Industries
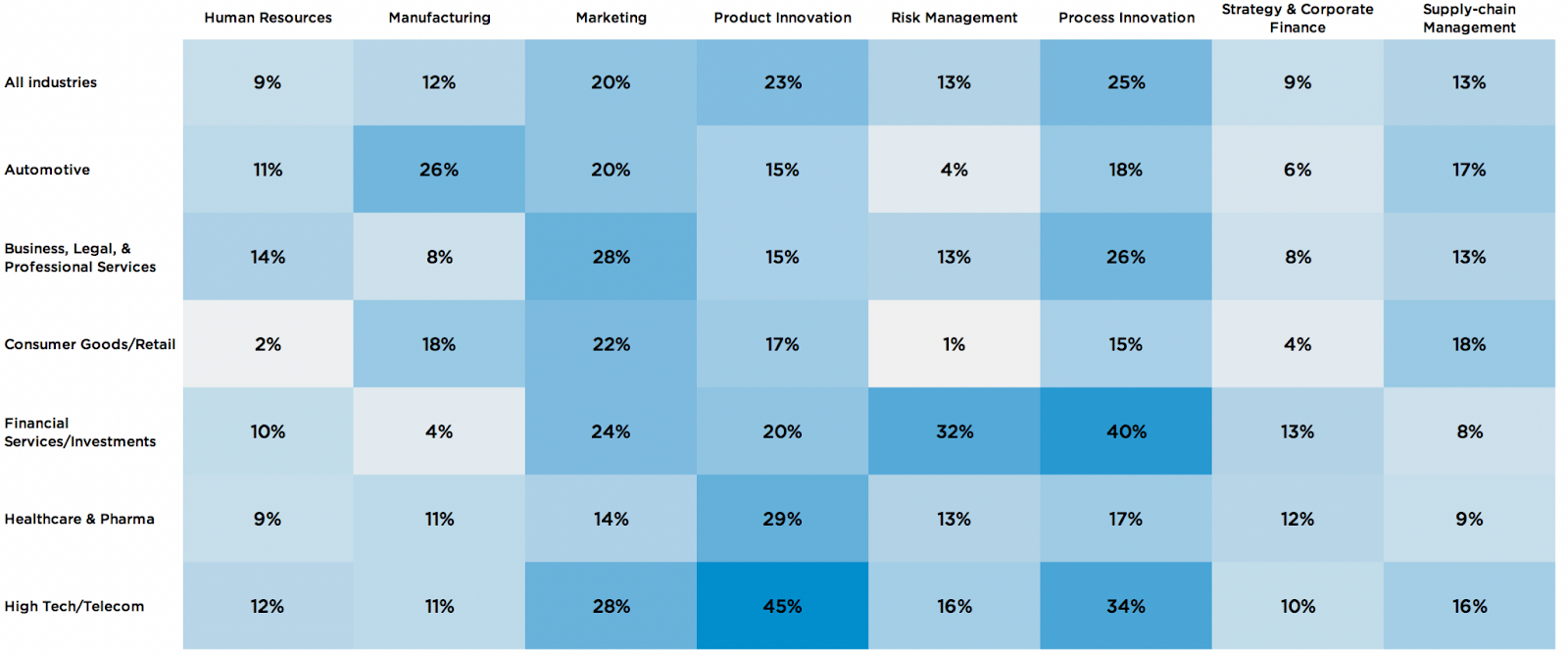
In investments, AI applications have matured and gained reliability. AI's impact on investment management encompasses the development of forward-looking investment strategies and the creation of tailor-made and diversified portfolios. Deloitte highlights that institutional investors embracing AI in their investment processes experience an average productivity increase of 14%, while assets under management (AuM) grow by over 8%, and revenues by 7%. These benefits extend across traditional institutional investors, family offices, private banks, and wealth managers.
The ‘tech factor’ benefit institutional investors, banks, advisors, and family
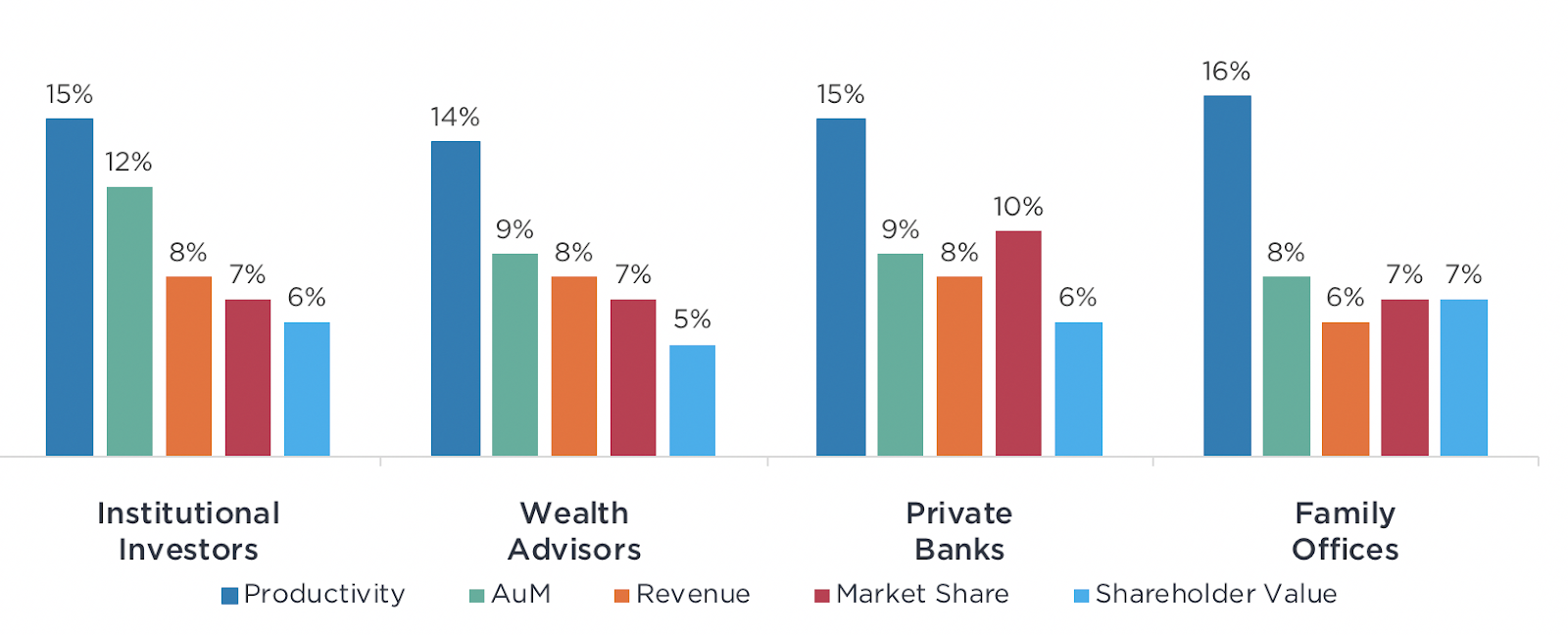
AI sets a new gold standard for creating forward-looking and robust investment strategies. It addresses time-consuming and mundane tasks, identifying investment signals from the noise in financial markets. It's no wonder that Asset and Wealth Managers are increasingly keen to integrate AI into their investment processes, given its potential to drive growth in AuM, productivity, revenues, and more.
Unlocking Insights with Generative and Analytical AI
In investments, two distinct yet complementary AI branches have emerged: analytical AI and generative AI. To understand their significance in investment decision-making, it's essential to grasp their fundamental differences and how they are leveraged in order to guide investment decisions.
Analytical AI: Uncovering Valuable Insights
Analytical AI is the technology that harnesses Machine Learning techniques to delve into data, uncover hidden insights, identify novel patterns, and establish relationships within datasets. This form of AI operates on principles deeply rooted in statistics and mathematics. Some common methodologies employed by analytical AI include Linear Regression, Neural Networks, Decision Trees, and Random Forests.
Analytical AI scrutinises vast volumes of historical and real-time data, aiming to extract meaningful information. In the financial landscape, the role of analytical AI can be exemplified by MDOTM's investment platform, Sphere. Sphere, with its analytical capabilities, meticulously analyses millions of historical, market, and macro data points. This thorough and advanced analysis unveils hidden signals within the noise of data, so then investment professionals can guide accordingly their investment decisions.
Generative AI: Enhancing Explainability
Generative AI has a completely different architecture than analytical AI. Generative AI models learn the patterns and structure of their input data, and then generate completely new data that has similar characteristics. Generative AI also includes a “layer” that facilitates an ongoing human interaction with the technology to produce novel outputs. Generative AI thrives on the power of creativity and imagination, and it often operates in response to specific prompts or requests provided by humans. Unlike analytical AI, which sifts through existing data to uncover valuable insights, generative AI generates entirely new content based on the input it receives.
For example, consider Midjourney, an AI-powered image generation tool. Users interact with Midjourney by providing textual or visual prompts, such as a description of the image they wish to see or even an existing image as a reference. Generative AI like Midjourney then creates a unique image based solely on the user's input.
Applying Generative and Analytical AI to Investment Decisions
Analytical AI excels at sifting through vast datasets, historical market data and economic indicators to identify trends and correlations that humans might traditionally overlook. It provides investment professionals with data-driven insights, risk assessments, and portfolio optimisation strategies. By automating complex data analysis, it frees up human experts to focus on higher-level strategic decisions.
Generative AI plays an important role in ensuring AI explainability in investments. It accomplishes this by generating comprehensive and clear explanations for the analytical output of AI. When investment professionals or clients receive AI recommendations, generative AI can provide detailed reports that break down the rationale behind each recommendation. These explanations shed light on why certain investment choices are made, helping to understand the technology’s reasoning, and ensuring AI explainability.
The Nexus Between Generative and Analytical AI
In investment management, the relationship between generative and analytical AI emerges as a powerful tool, enhancing the way investment decisions can be made and understood.
Analytical AI, with its proficiency in data analysis, serves as the foundation of this partnership. It's the engine that processes vast datasets, examining historical market data, and economic indicators. Analytical AI has the capacity to uncover intricate trends, correlations, and patterns. It provides investment professionals with invaluable data-driven insights, risk assessments, and strategies for optimising investment portfolios.
Generative AI, on the other hand, plays a role in ensuring transparency and explainability in investment decisions. It works with human interaction and operates in response to specific prompts or queries. This aspect of generative AI is particularly significant when applied to investments, as it bridges the gap between the analytical output of AI and the human understanding of the technology’s reasoning.
The synergy between these two forms of AI is most evident with regards to AI explainability. Investment professionals often seek to understand the rationale behind AI-generated investment inputs. While analytical AI can pinpoint trends and suggest portfolio adjustments, generative AI steps in to provide comprehensive explanations. When an investment decision is made based on analytical AI's insights, generative AI can create detailed reports that explain the reasoning behind each recommendation. These reports serve as a critical link between the intricate analyses performed by analytical AI and the decision-makers involved. They break down complex data into understandable narratives, allowing investment professionals and clients to grasp why particular investment insights have been provided.
For instance, if analytical AI recommends a shift in portfolio allocation, generative AI can produce an explanation outlining the underlying factors, such as recent market developments, economic indicators, or company performance metrics. This interpretation empowers investment professionals to make informed decisions and communicate the rationale behind their choices effectively.
The nexus between generative and analytical AI is reshaping the modern approach towards investment management. Analytical AI's data-driven capabilities are harnessed to uncover hidden insights and optimise portfolios, while generative AI ensures these insights are translated into clear and comprehensible explanations. Together, they empower investment professionals with a data-driven precision and human-centric understanding.
AI Integration: From Research to Maturity
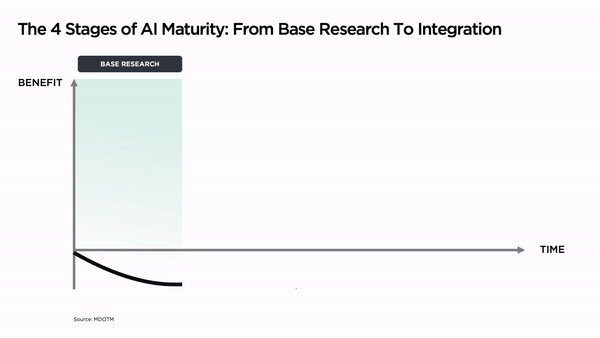
As we've explored earlier, analytical AI excels in data analysis, uncovering insights, and optimising portfolios, while generative AI ensures AI's understandability by providing comprehensive explanations for its decisions. In order to comprehend how these technologies are being integrated into the investment process of numerous financial institutions, we will delve into each stage of our AI maturity model, shedding light on the journey from Base Research up to the actual Integration. To illustrate our research, we will also draw upon Gartner's AI maturity model, which segments companies into five levels of maturity regarding their use of AI.
1. Base Research: Laying the Foundation for AI
The Base Research stage aligns with the initial awareness of AI's potential (Level 1 in Gartner's model). During this phase, organisations recognise the power of AI but have yet to harness it effectively. MDOTM refers to this phase as the Base Research stage. It is where the groundwork is laid, where we invest in understanding the AI landscape, its capabilities and limitations.
2. Applied Research: Experimentation and Learning
As organisations advance to Gartner's Level 2 - Active, they begin to experiment with AI in practical settings, transitioning from mere awareness to active experimentation. This phase corresponds to the Applied Research stage, where AI applications move from ideation to practical applications. When AI is still in its developmental stages, it starts to demonstrate practical value through experimentation and learning.
3. Custom Applications: Tailoring AI to Specific Needs
At Gartner's Model Level 3 - Operational, companies have embraced Machine Learning, deploying it in daily operations. Here, AI is tailored to meet specific requirements, ensuring that solutions align precisely with clients' objectives. Here, the technology begins to deliver more substantial benefits compared to its earlier stages, providing clients with highly customised and tangible value.
4. Integration: AI as an Integral Part of Investments
Finally, at Gartner's Level 4 - Systemic, companies fully integrate AI into their processes and infrastructure. In this stage, the technology becomes an integral part of investment decision-making. It actively shapes investment strategies and provides forward-looking, timely insights.
The seamless interaction between generative and analytical AI, coupled with their progression through the stages of AI maturity, leads to a future where AI-driven investment management is not just a choice but a necessity to have a competitive edge in the financial industry. This approach empowers investors to make informed decisions, optimise portfolios, and thrive in an ever-evolving landscape. As the financial industry continues to evolve, the partnership between generative and analytical AI will play a pivotal role in ensuring AI's maturity, explainability, and effectiveness in investment management.
To discover more about how to integrate AI into your investment process, reach out to our experts
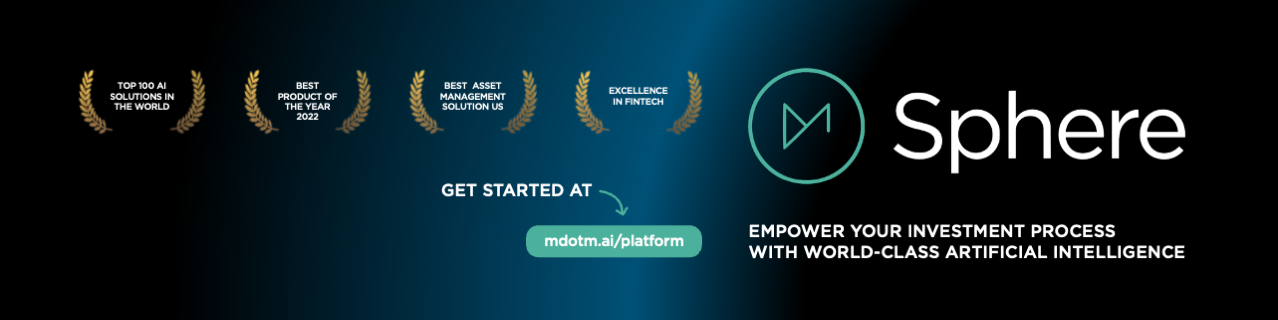